Abstract
In recent times, there have been many advances in anomaly detection for computer vision applications. Despite this, the problem of anomaly detection on any vehicles undercarriage remains very challenging for two main reasons:
First, the data domain for a vehicle undercarriage is very unique; there is no publicly available data set, and its not readily available online.
Second, there is no dataset of threats to be detected, which can appear in any place or form (explosive devices, weapons, contraband etc). Essentially, this is a semi-supervised anomaly detection problem, where the anomaly class does not exist in the dataset.
In this presentation, we will describe the steps we took to solve this problem, including deep learning models for representations of vehicles, similarity metrics, segmentation, anomaly detection, and how all these models are combined into a singular system that analyzes a vehicle in just few seconds. We will also show how models trained for security purpose have great value in the automotive industry, whereby in using similar systems we can detect various types of mechanical problems and damages to the exterior of any vehicle. By using such technologies for anomaly detection in vehicles in automotive/civilian context, we can enable and streamline predictive maintenance practices, and consequently ensure safe and reliable mobility.
Bio
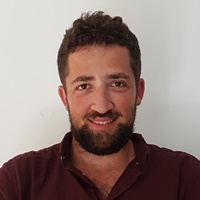
Deep Learning Team Lead
UVeye
Ilya joined UVeye to lead the development of the deep learning algorithms team.
Ilya is responsible for developing the visual threat assessment and anomaly detection algorithms in Uveye, providing state of the art vehicle inspection capabilities for both the security and automotive industry.
Prior to his current position, Ilya was responsible for developing deep learning algorithms for Robotics visual perception and decision-making technology.
Ilya holds a B.Sc. in Electrical Engineering from Ben-Gurion university.