Senior Algorithm Developer, Machine Vision Group
Applied Materials
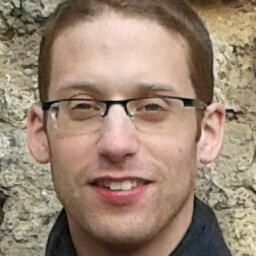
Bio:
Danny Ravid joined Applied Materials’ machine vision algorithms group in 2012, and since then has been working on development of computer vision algorithms combining state of the art procedures for image processing and machine learning.
Danny holds B.Sc and M.Sc in Electrical Engineering, both from Tel-Aviv University.
Title:
Multi-Modal Gradient-Based Registration between CAD Data and SEM Images
Abstract:
Advanced microscopic imaging tools, such as a scanning electron microscope (SEM), are often used in the semiconductor industry for various wafer-inspection related tasks which are performed during the process of microelectronic devices fabrication.
Registration between CAD (computer aided design) data and a microscopic image containing the actual structures as printed on the wafer, is a powerful ability, useful for various applications: SEM image segmentation, which is then used for detection and classification of defects; Accurate measurements between fabricated structures; CD(critical dimension) measurements; Alignment between various tools and coordinate systems, and more.
However, this registration task is a non-trivial one, since it involves the matching of two different modalities, namely CAD (polygons) and SEM (image pixels). Moreover, the structures seen in SEM images can look actually quite different from the designed “ideal” CAD, due to the various fabrication process steps that the wafer undergoes before the image is taken, in addition to challenging imaging conditions (such as low resolution or SNR). Therefore, common registration techniques do not work well for this task.
In this work, we present a robust multi-modal registration algorithm between CAD and a microscopic image. The method first applies some transformations to the CAD in order to make it look as similar as possible to the actual image (synthetically imitating the effect of the various process steps), then performs a registration based on complex gradient correlation between the two modalities.
This registration approach has shown to achieve highly accurate and robust results for the described task, and to work well under challenging scenarios.